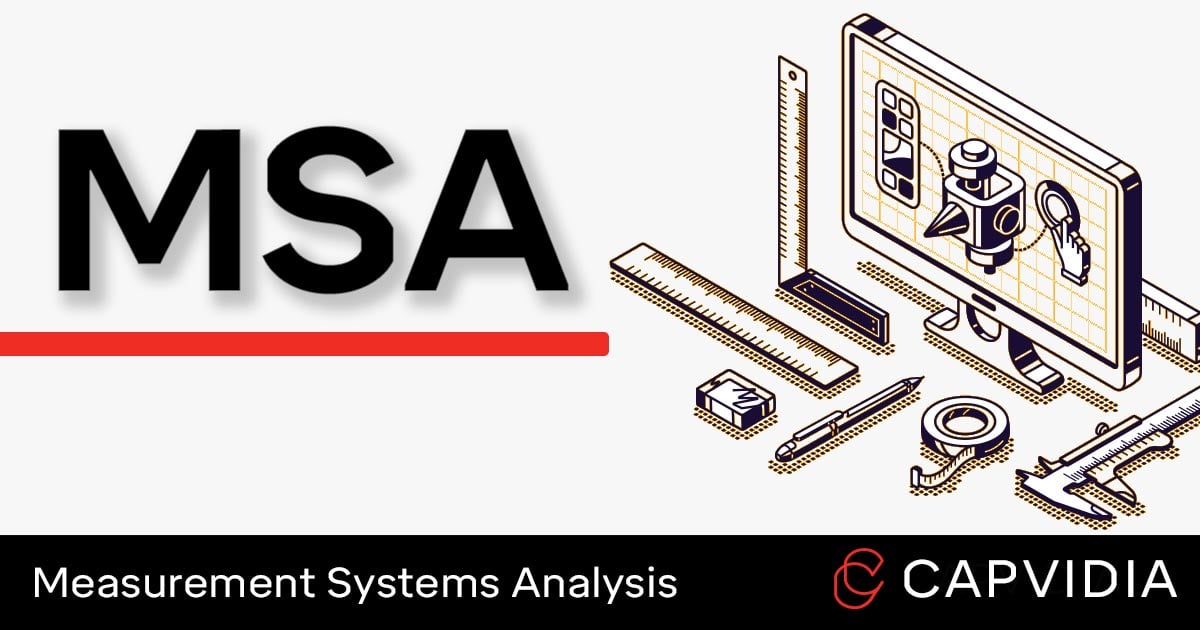
- MSA is a statistical process that identifies the integrity of the measurement system itself.
- MSA proactively looks for variability within processes and products that fall outside acceptable tolerances.
- MSA aims to ensure the tools and processes are accurate and precise.
Table of Contents:
- What is MSA?
- Why is MSA important?
- When is MSA necessary?
- How the MSA process works.
- Digitizing the MSA process
- Pundit: Measurement uncertainty simulation
What is Measurement Systems Analysis (MSA)?
Measurement Systems Analysis (MSA) is a statistical process used to assess the amount of variation in the measurement process introduced by the measurement system itself.
In the manufacturing industry, variability within the process and product is inevitable.
The role of manufacturing quality ensures that the process and product conform to specifications and that any variability falls within acceptable tolerances through measurement and data collection.
But how do you know if your measurement is accurate?
What if the measurement equipment (gage) is inaccurate or incorrectly calibrated? What if the operator carrying out the work is inconsistent? What if there’s a big difference in measurement values between two operators for the same part?
Then, all measurements and data would be flawed, leading to a flawed process and flawed product.
MSA examines these three crucial details :
- Measuring device: How much variation exists within the tool & equipment.
- Personnel: How consistently do individuals perform the measurement?
- Measurement method: How reliable is the measurement process?
MSA is part of the five Core Tools for effective quality management with APQP, PPAP, FMEA, and SPC being the other core tools.
Measurement System consists of the following:
- Equipment and tools: Gages, testing instruments, etc.
- Operator: Training, assumption, fatigue, etc.
- Standard operating procedure.
- Environment.
Why is MSA important?
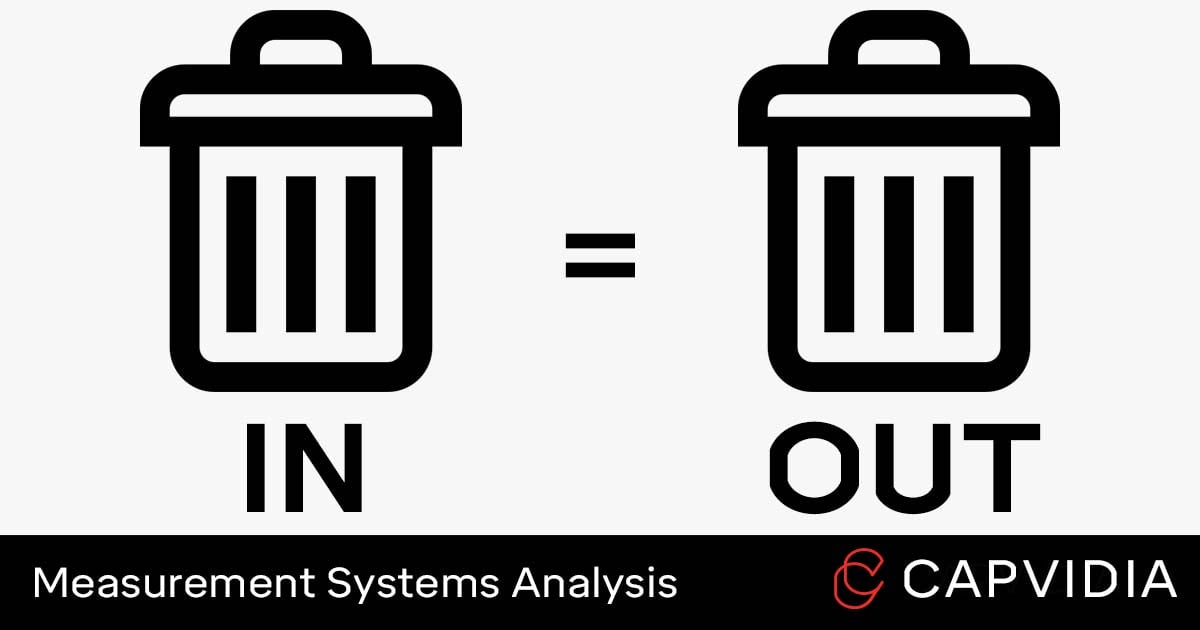
MSA looks for the classic case of garbage in, garbage out.
Accurate measurements lead to accurate data lead to the best possible decisions for designing and manufacturing a product.
However, faulty measurements lead to faulty data and poor decisions for designing and manufacturing a product.
The results?
Expensive scrap, rework, defects, retesting, and recalls, which is known to be 29X more expensive for corrective action in the operations phase compared to planning phase.
Quality decisions come from quality data.
MSA confirms the integrity of your measurement systems.
5 Benefits of MSA:
- Prevents For Want of a Nail or GIGO situation.
- Identifies and reduces measurement errors.
- Improves process reliability, quality, and safety.
- Confidence in data to make better decisions.
- Increased customer satisfaction.
When is MSA necessary?
MSA is necessary in various scenarios within an organization's quality management and process control activities. For example:
- Changes in the manufacturing process.
- New measurement system.
- Routine checkup to avoid drift.
- Post repair and maintenance.
- Detected quality issues.
- Supplier qualification & monitoring.
How MSA process works.
The MSA confirms the fidelity of measurement variation. Measurement variation is defined by accuracy and precision.
Accuracy
Accuracy is the average measurement close to a reference value (master sample).
Accuracy includes:
- Stability: accuracy over time.
- Linearity: accuracy throughout the measurement range.
- Resolution/Discrimination: accuracy with varying levels of detail.
- Bias: difference between observed value and reference value.
Let's use a bathroom scale as an example.
Stability: Assuming your body weight remains the same, will the reading be the same today as tomorrow or a week from now?
Linearity: Assuming heavier or lighter family members weighed themselves, would the measurement scale appropriately?
Resolution: How detailed is the measurement from a mechanical scale vs a digital scale?
Bias: What if the scale’s resting point starts out at 5 pounds instead of 0?
Precision
Precision is a consistent measurement each time or the closeness of two or more measurements to each other.
Precision includes:
- Repeatability: Getting the same measurements using the same tool, same part, and same person.
- Reproducibility: Getting the same measurements using the same tool, same part, but different person.
Let’s use a cooking recipe in this example.
Repeatability: Could the same person use the same recipe and the same equipment to produce a consistent dish repeatedly?
Reproducibility: Could a different person take the same recipe and same equipment and produce that same dish repeatedly?
Gage Studies
Gage studies are MSA tools used to understand measurement uncertainty due to possible errors in measuring accuracy and precision.
Type 1 Gage (Accuracy)
A Type 1 Gage study identifies any deficiencies in the measurement system.
One operator measures a reference part multiple times, usually over 50. The mean of all measurements is then subtracted from the reference part’s value producing the bias.
If measurements fall outside the acceptable tolerance range, then there may be a problem with gage accuracy.
Gage R&R (Precision)
A Gage R&R (Repeatability & Reproducibility) study estimates the combined variation caused by repeatability and reproducibility errors.
It determines two important aspects: how much variation is due to equipment (gage variability) and how much variation is due to personnel (operator variability).
The end results are calibration or replacements to gages and measurement tools and updated training and procedures for operators.
There are two types of Gage R&R studies: Gage R&R for variable data (infinite values) and Gage R&R for attribute data (specific values).
Here are the recommended conditions for both:
Recommended Gage R&R study for variable data:
- Number of operators (appraisers): Minimum 2. Recommended 3 or 4.
- Number of parts: Minimum 10.
- Number of trials: Minimum 2. Recommended 3.
- Total measurements: 90.
- To be measured in random order.
- Operators should not be aware of measured value while measuring same part again to avoid knowledge bias.
- Operators should use the same method for measurement.
Recommended Gage R&R Study for attribute data:
- Number of operators (appraisers): Minimum 2. Recommended 3.
- Number of parts: Minimum 30. Recommended 50.
- Evaluate all parts by Expert Operator for correct decisions.
- Each operator should assess (pass, fail) all the parts 3 times independently and in random order.
- Record results.
- Calculate scores for Effectiveness, Miss Rate, False Alarm and Kappa.
- Effectiveness: number of correct decisions
- Miss Rate: reject part evaluated as pass by Operator
- False Alarm: Pass part evaluated as rejected by Operator
- Kappa: Level of agreement between two operators rating the same data.
The Gage R&R study results in a score to be compared against the reference value produced by the master sample.
Below are the guidelines for judging acceptability.
Acceptable criteria for Gage R&R Study for variable data:
- Less than 10%: Acceptable.
- 10 to 30%: May be acceptable depending on relative importance.
- More than 30%: Unacceptable.
Acceptable criteria for Gage R&R Study for attribute data:
Decision for Operator | Effectiveness | Miss Rate | False Alarm |
Acceptable | ≥90% | ≤ 2% | ≤ 5% |
Minimally acceptable but needs improvement | ≥80% | ≤5% | ≤10% |
Unacceptable | ≤80% | ≥5% | ≥10% |
Kappa Value | ≥75% |
Typically, if a repeatability value is more considerable compared to the reproducibility value, then it’s possibly a gage error. If the reproducibility value is more substantial compared to the repeatability value, then it’s most likely operator error.
Possible Causes of Measurement System Error
Stability:
- Instrument needs calibration.
- Worn instrument, equipment or fixture.
- Normal aging or obsolescence.
- Poor maintenance: air, power, hydraulic, etc.
- Worn or damaged master, error in master.
- Improper calibration or use of setting master.
- Poor quality instrument: design or conformance.
- Different measurement method.
- Distortion (gage or part).
- Environment: temperature, humidity, vibration, cleanliness, etc.
- Violation of an assumption, error in applied constant.
- Application: part size, position, operator skill, fatigue, observation error (stability, parallax).
Linearity:
- Instrument needs calibration.
- Worn instrument, equipment or fixture.
- Poor maintenance: air, power, hydraulic, etc.
- Worn or damaged master, error in master.
- Improper calibration or use of setting master.
- Poor quality instrument: design or conformance.
- Instrument design or method lacks robustness.
- Wrong gage for application.
- Different measurement method.
- Distortion (gage or part) changes with part size.
- Environment: temperature, humidity, vibration, and cleanliness.
- Violation of an assumption, error in applied constant.
- Application: part size, position, operator skill, fatigue, observation error (readability, parallax).
Bias:
- Instrument needs calibration.
- Worn instrument, equipment or fixture.
- Worn or damaged master, error in master.
- Improper calibration or use of setting master.
- Poor quality instrument – design or conformance.
- Linearity error.
- Wrong gage for application.
- Different measurement method.
- Measuring wrong characteristic.
- Distortion (gage or part).
- Environment: temperature, humidity, vibration, and cleanliness.
- Violation of an assumption, error in applied constant.
- Application: part size, position, operator skill, fatigue, observation error (readability, parallax).
Repeatability:
- Within-part (sample): form, position, surface, finish, taper, sample consistency.
- Within-instrument: repair, wear, equipment or fixture failure, poor quality or maintenance.
- Within-standard: quality, class, wear.
- Within-method: technique, position, lack of experience, manipulation skill or training, feel, fatigue.
- Within-environment: short-cycle, fluctuations in temperature, humidity, vibration, lighting, cleanliness.
- Violation of an assumption: stable, proper operation
- Instrument design or method lacks robustness, poor uniformity.
- Wrong gage for application.
- Distortion (gage or part), lack of rigidity
- Application: Part, size, position, observation error (readability, parallax)
Reproducibility:
- Between-parts (sample): average difference when measuring types of parts A, B, C, etc., using the same instrument, operators, and method.
- Between-instruments: average difference using instruments A, B, C, etc., for same parts, operators, environment.
- Between-standards: average influence of different setting stands in the measuring process.
- Between-methods: average difference caused by changing point densities, manual versus automated systems, zeroing, holding or clamping methods, etc.
- Between-operators: average difference between operators A, B, C, etc., caused by training, technique, skill, and experience.
- Between-environment: average difference in measurements over time 1,2,3 etc. Caused by environmental cycles; this is the most common study for highly automated systems in product and process qualifications.
- Violation of an assumption in the study.
- Instrument design or method lacks robustness.
- Operator training effectiveness.
- Application: part size, position, observation error (readability, parallax).
Source: Measurement Systems Analysis 4th Edition by AIAG (Automotive Industry Action Group)
Digitizing MSA workflows
MSA produces valuable data for immediate but also long-term improvements.
Most data is viewed as pass/fail and often discarded despite an era in which digital is king and data is the new oil.
A model-based definition (MBD) introduces data back into the ecosystem for improved designs, improved parts, and improved operations done quicker, cheaper, and more efficiently.
MBD is a growing industry practice of having the 3D CAD model be the “single source of truth.”
This means the CAD model along with semantic product and manufacturing information (PMI) can drive the MSA, APQP, PPAP, and SPC reports along with containing GD&T, bill of materials, notes, engineering change orders etc. for an improved method of inspection generation and automating other manual processes.
The power of MBD-based approach is the human and machine readability factor allowing for interoperability between machines and software with less reliance on human process and interpretation.
MBD Benefits:
- Automated: No need for human transcription or interpretation.
- Build and optimize a reliable and repeatable process.
- Data mapped back to the single source of truth.
- Start of the digital transformation from design to manufacturing.
- Valuable data to mined for analytics and business insights.
Pundit: Measurement Uncertainty Simulation
Gage R&R alone isn't enough for a complete MSA, because it is possible to have results that are repeatedly and reproducibly incorrect aka the data looks "good."
Measurement bias must also be included to ensure the accuracy of the measurement system.
Pundit captures both repeatability and bias measurements to limit the risks and also the financial impact of parts that meet spec but do not work of fit.
What Pundit does:
- Reduce measurement uncertainty and improve process control.
- Calculate task-specific measurement uncertainties for CMM measurements.
- Capture measurement repeatbility and bias.
- Not reliant on any physical part, provides quick insights before cutting metal.
- Pay with different measurement strategies in order to achieve lower measurement uncertainties.
Pundit: Smart CMM Inspection Simulation Software.
Have other questions about measurement uncertainty?
Contact us and we'll glad to help answer questions about MSA and preventing measurement errors.